Exploring the pitfalls of algorithm-driven ad platforms in lead generation
Boomer Marketing, Digital Direct Marketing, Marketing Analytics, Medicare Marketing, Response Marketing, Trends and POVOn March 4, Microsoft took its own version of Performance Max out of BETA, making it available to all advertisers. It joins Google’s Performance Max, Meta’s Advantage+, and others in the trend of leaning fully on algorithm-based digital ad platforms.
Google’s P-Max originally debuted back in November 2021. It utilizes machine learning to optimize bids and drive conversions across its entire ad network, including Google Search, Display, Gmail, DemandGen, and YouTube. Microsoft’s P-Max reaches a smaller but still targeted audience across Bing Search, Yahoo, AOL, and Linkedin. Meta’s Advantage+ reaches audiences across Facebook, Instagram, and Messenger.
Benefits of algorithm-based marketing platforms
Before diving into the potential pitfalls of AI and machine learning (ML) algorithm-based marketing, let’s first acknowledge the undeniable advantages AI brings to the table in terms of marketing.
First and foremost, ML algorithms excel at processing large amounts of data. They can easily identify patterns and trends in user behavior. Additionally, because they can continuously analyze performance based on conversion objectives, they can adjust bids in real-time. This ensures that the budget is always directed toward the most effective placement at any given time.
But despite the benefits, there are very real drawbacks of ML algorithms that can result in your campaigns going off the rails. I highlight some of the biggest potential pitfalls and how to avoid them below.
Lack of human control
One of the biggest concerns with ML-driven ad platforms is their lack of transparency. Unlike traditional digital media campaigns, where marketers have full control over targeting, bidding, and creative assets, AI platforms often operate as a bit of a “black box.” Marketers must relinquish a certain degree of control, relying on algorithms to make decisions about ad placement, optimization, and budget allocation. This lack of insight makes it challenging to identify areas for improvement and optimize campaigns for lead quality.
For instance, the platform within Meta’s Advantage+ tailored ad campaigns’ attempts to cast a wider net by default and doesn’t allow you to fully restrict your audience based on age. You can input desired age ranges, and they’ll be prioritized — but other ages won’t be fully excluded.
This can be problematic for a Medicare audience, which is typically 64+ years old. Yet, if you choose an Advantage+ campaign, “minimum age” won’t go above 25 years old. In a similar vein, there’s no real ability to exclude specific audiences (e.g., current or past members, or specific audiences you don’t want to target).
Questionable lead quality
The concept of lead generation presents a unique challenge for AI algorithms. While AI excels at optimizing for conversions in ecommerce — where a purchase signifies success — lead quality is a more nuanced concept. Lead generation is typically a “top funnel” activity, aiming to attract potential customers who may be early in the buying journey. AI platforms currently lack the ability to independently differentiate between high-quality and low-quality leads.
If marketers don’t provide clearly defined conversion goals and detailed conversion data, AI platforms tend to treat all leads equally. This risks the platforms potentially prioritizing low-quality (and even spammy) leads from unreliable sources or bot traffic. This can lead to a surge in unqualified leads and overwhelm sales teams. For example, if a campaign using P-Max has a significantly lower cost per lead (CPL) compared to a standard campaign, it’s a clue that P-Max is errantly optimizing low-quality leads.
By understanding and addressing the challenges posed by algorithm-driven ad platforms, marketers can position themselves to make informed decisions, optimize campaigns effectively, and achieve long-term success.
Marketers need to be extremely vigilant about monitoring lead quality with sales teams and providing feedback to the platform in order to refine targeting and prioritize high-value leads. Some examples of feedback you may consider providing: everyone who has converted on your landing page/website, email lists, and plan members (to use as exclusions within acquisition campaigns).
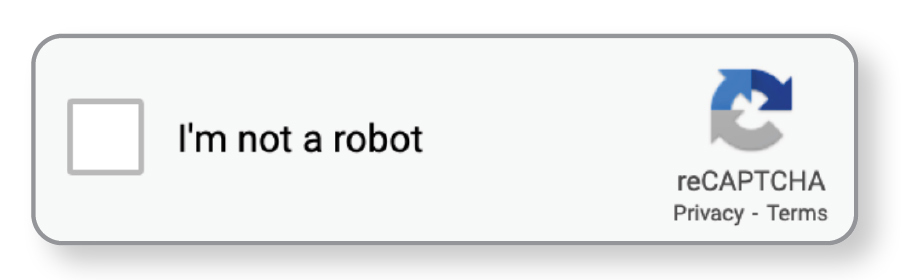
Additionally, if your leads are derived from web-based form submissions, you may wish to implement a CAPTCHA solution in an attempt to block bot traffic from submitting your forms.
Lastly, it’s worth pointing out that P-Max can even choose a URL for you. It does this based on where it believes your traffic is most likely to convert. This option is checked by default. For a lead generation campaign, this can be problematic if the algorithm attempts to funnel traffic to a page or website that doesn’t have the appropriate message, isn’t properly branded, and/or doesn’t have the same conversion actions.
Limited reporting
While P-Max provides insights on overall campaign performance, it doesn’t provide breakdowns by asset or placement (Search, Display, etc.). Data is simply highlighted as “cross-network” within GA4. This makes it challenging to analyze campaign performance at an individual placement level — a feature that has been long utilized by traditional digital media campaigns.
Skewed emphasis on branded queries
P-Max is notorious for bidding aggressively on branded search terms. This can be a challenge for those with a heavy search engine optimization (SEO) presence for branded terms. In the insurance industry, this can result in wasted spending on existing members who may be looking for a member services phone number or other member-related information that can be freely found.
Fortunately, Google has campaign-level brand exclusions, which can be tailored to function like its traditional account-level negative keywords, which are found within traditional search campaigns. Branded queries that are clearly targeting members can be added here.
Despite some of the potential for overlap discussed above, it’s important to note that P-Max can be effectively used in conjunction with an established paid search campaign. If a search query exactly matches keywords (of any match type) within your account, the search campaign will be prioritized over P-Max.
Unusual creative combinations
Unlike standard ad campaigns, P-Max and Advantage+ campaigns allow you to upload multiple images, headlines, and description lines. From there, the algorithms decide how to use these combinations. At times, this creative freedom can result in illogical (or not completely brand-friendly) ads. These platforms can even attempt to make a generic video so they can include video within their ad inventory.
For Medicare campaigns, this can be potentially problematic due to the requirements for ad campaigns established by the Centers for Medicare & Medicaid Services (CMS). Fortunately, Google can be provided with some “guardrails” to tighten controls on algorithms.
Saying “Yes” to all recommendations
Google’s recommendations tend to be automated as a result of analyzing millions of accounts. However, all these platforms tend to discount the nuances of your specific campaign — and your organization. Often, these recommendations are successful at one thing — spending your budget — but not always with a significant increase in conversion performance. While it’s generally a good idea to continually test, it’s also okay to say “no” to automated recommendations within these platforms.
Budgetary burdens
Transitioning to AI-driven ad platforms often necessitates an increased budget. With ads appearing in more places, there’s a need to effectively test and optimize performance across each platform. Testing an unproven platform can present a significant cost consideration for your organization, especially for a smaller business with limited marketing resources.
So, where is the industry headed?
Despite all the above potential pitfalls, Google, Microsoft, and Meta are hedging their bets that marketers will opt for return on ad spend (ROAS) over transparency and control. Due to privacy concerns and the loss of third-party cookies, I believe it’s only a matter of time until all ad platforms are forced to move in the direction of algorithmic marketing platforms. As a result, it’s imperative for marketers to strike a balance between leveraging the advantages of automation and mitigating the pitfalls.
By understanding and addressing the challenges posed by algorithm-driven ad platforms, marketers can position themselves to make informed decisions, optimize campaigns effectively, and achieve long-term success.
Do you have questions about AI and algorithm-driven ad platforms? Reach out to us today!